Forecasting Long Haul Truckload Spot Market Rates
Research publications
Research Team
Contact Team
The project aims at forecasting long haul truckload spot market rates in the continental USA for the near future. Accurate forecasting of transportation costs is a key step in logistical planning. It helps buyers and sellers of transportation services make better decisions at all stages of a supply chain. Short term spot rate forecasts can help in making operational decisions, estimating budgets for shippers and cash flow for carriers.
The first part of the analysis is at a 3-Zip origin region level. Making frequent forecasts for volatile time series such as truckload spot rates comes with its challenges. We solve the problem using four models: Naive, Moving Average, Auto-Regressive Integrated Moving Average, and Feed-Forward Neural Networks. An important aspect of the truckload spot market is the periodic cycles of soft (decreasing market rates) and tight (increasing market rates) markets. Such changes in the time series, or concept drift, make old forecasting models irrelevant. Thus, we employ concept drift handling techniques to re-train the models regularly with new information to account for changes that may appear in the underlying data structure over time. Finally, we draw inferences from the MAPEs of the models and comment on their merit.
The second part focuses on the national level. First, we check if the weekly spot rates time series is a Random Walk process. In this case a Naïve forecast is better than other auto-regressive time series models and thus we use it as our baseline forecast. We then used exogenous economic indicators as inputs to a Linear Regression model, fit using Elastic Net Regularization, to check if there are leading indicators for truckload spot rates. To account for changes in market cycles as described above, we also used two implicit and one explicit concept drift handling methods to retrain our forecasting models. Finally, we comment on the relative performance of the models by comparing the MAPE of the forecasts. We also discuss how explicit detection of concept drift provides useful information on changes in the market cycle for the stakeholders.
Research Publications
- Forecasting short term trucking rates, SCM Thesis, 2018
- Characterization and short term forecasting of the US long haul truckload spot market, MST Thesis, 2019
- Forecasting Long Haul Truckload Spot Market Rates, Working Paper, 2019
Research Team
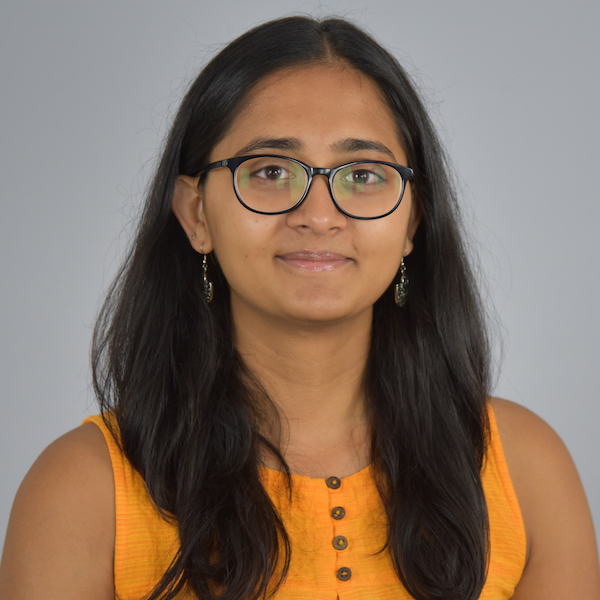
Shraddha Rana
Research Assistant

Dr. Chris Caplice
Co-Director
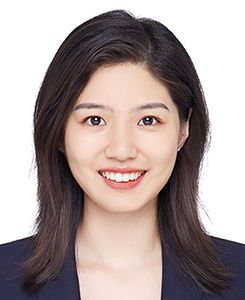
Dr. Xiwen Bai
Research Contributor
Tsinghua University